Algae are terrific at fixing carbon. Like plants, they absorb large quantities of carbon dioxide from the atmosphere and convert it into sugars via photosynthesis. Plus, the slimy green stuff grows faster than trees, and can grow on land and in water unsuitable for food crops. All these are reasons why algae are considered an important tool to address climate change.
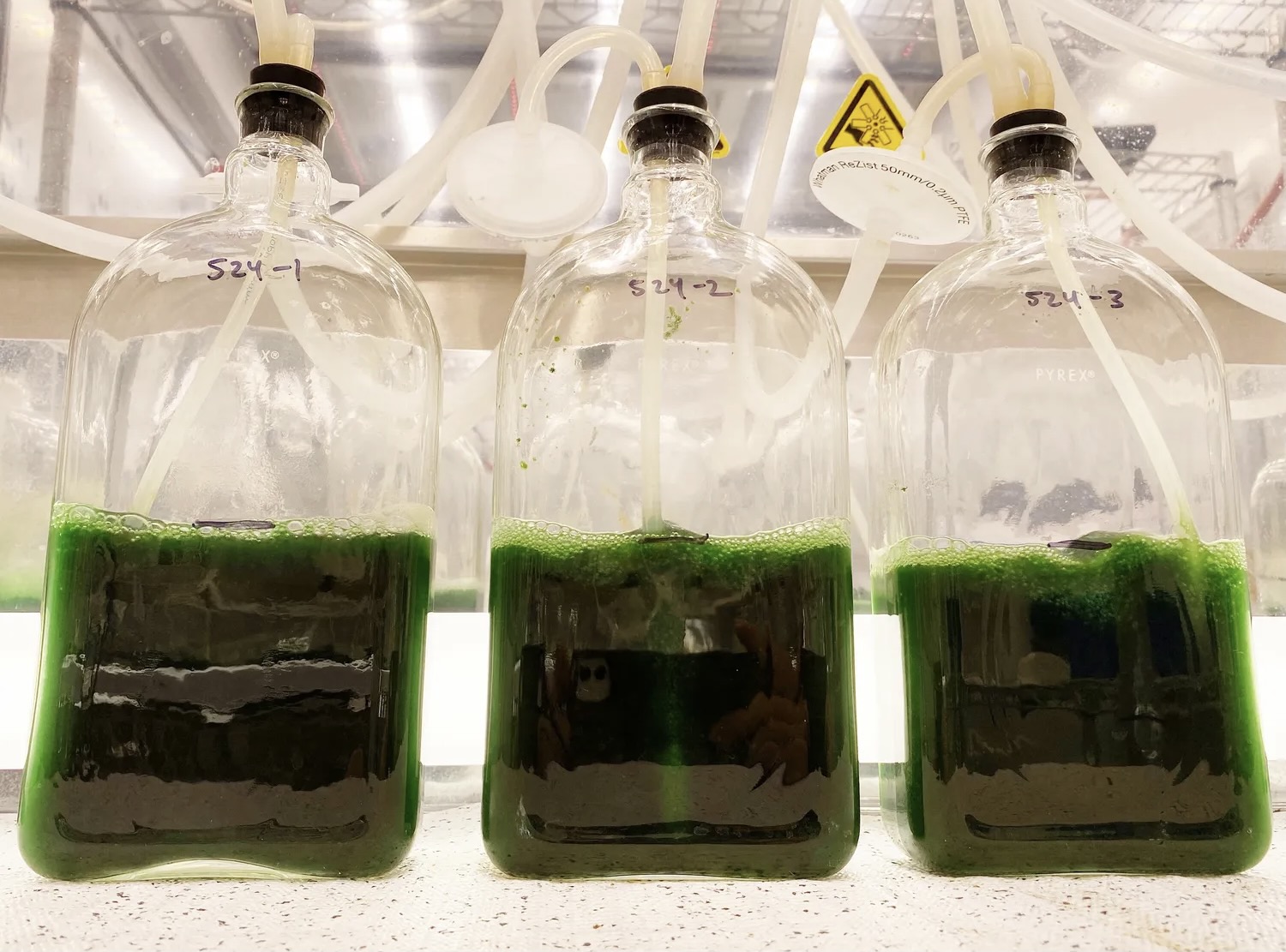
That is, until you try to cultivate algae at large scales. Producing large enough amounts of algae to be cost-effective has proven immensely challenging and kept algae biofuels from reaching the market. But now researchers have tapped into artificial intelligence to grow algae at a record pace that could bring down algae fuel cost.
By using machine learning to precisely tune the growth of blue-green algae, also known as cyanobacteria, the Texas A&M University team is able to produce over 43 grams per square meter a day in an outdoor experimental setup. That’s about twice the 25 grams/m2/day target recently released by the U.S. Department of Energy. It’s “absolutely without any question the highest reported outdoor algae growth speed and productivity,” says Joshua Yuan, professor and chair of synthetic biology and renewable products at TAMU.
Their process has the potential to bring the algal biofuel price to below $5 per gallon from today’s exorbitant $33 per gallon.
The new high yield would bring the algae selling price to US $309 per tonne ($281 per tonne), less than a quarter of the selling price of algae cultivated in 2019 using state-of-the-art open-pond methods. Yuan cautiously estimates that his team's process has the potential to bring the algal biofuel price to below $5 per gallon ($1.32 per liter) from today’s exorbitant $33 per gallon ($8.71 per liter). That's slightly higher than corn ethanol, which is $2 to $4 per gallon (53 cents to $1.06 per liter) and is “already getting competitive with fossil fuels in California, as we can link to carbon capture to bring carbon credits,” he says. “By combining carbon credit and lowering fuel price, we can be competitive with fossil fuels. Furthermore, this fuel price is competitive in overseas markets, like China, where fuel prices are much higher.”
Algae is already cultivated commercially for nutritional supplements, cosmetics, and animal feed. A decade ago, there was a surge of interest in algal biofuels: The sugars and oils produced by algae can be turned into ethanol, diesel, and jet fuel. But no one could come up with an economical way to do that and turn a profit, says Yuan. This led two of the largest algal biofuel companies, Solazyme and Sapphire, to shut down, and a third, Algenol, to switch to nutritional and cosmetic products.
In early February, the DOE announced $19 million of funding for projects that can boost the capacity of algal systems to capture carbon dioxide, as a way to both reduce carbon emissions and produce algae for biofuels and other products. “Theoretical algae yield is huge, much better than land-based plants, but there are a few major limitations,” Yuan says—namely, growing and harvesting algae.
In both closed reactors and open-pond systems, as algae grows and its concentration increases on the surface, it blocks light from reaching the cells underneath, slowing down growth. Plus, harvesting it is inefficient and costly. Traditional ways to separate algae from water such as centrifugation, filtration, and chemical flocculation, can make up as much as half of the total energy use and third of the total costs of producing algae.
The AI-powered semiautonomous algae-cultivation system that Yuan and his colleagues report in Nature Communications overcomes these issues. The researchers developed two machine-learning models. One predicts how, based on the light intensity falling on algae in water and the density of algal cells, light gets scattered and distributed through the cell mass. Unlike previous models, this one predicts light distribution in 3D. The second model predicts how this light availability affects growth rate. By combining the two models, Yuan says, they can calculate the highest algae concentration that does not produce too much shade, allowing algae to grow at maximum speed in changing light conditions.
“Once the AI tells us, we control the algae growth at this concentration,” he says. As soon as that concentration is reached, the researchers manually remove a part of the algae and add a growth medium. In the future, they plan to make the system completely autonomous by making the removal robotic instead of manual.
The researchers also found a way to make harvesting faster and cheaper. They engineered the algae to produce a chemical called limonene that makes the surface of the cells water-repellent. As a result, the cells try to get away from water and cluster together, eventually getting heavy enough to settle down at the bottom of the vessel and making them easier to remove.
The AI models can be adapted to different size setups and lighting conditions, and optimized for factors like nutrition and temperature. The TAMU team adapted the system for a 30-liter outdoor pond that they tested in September 2021. Yuan says that with a new $2 million grant from the DOE Office of Fossil Energy and Carbon Management, they plan to test the technology to capture carbon dioxide from the flue gases emitted at power plants owned by Southern Co.
AI-solutions company Hypergiant has developed a small bioreactor that also uses AI to optimize algae growth. Their modular reactor is built to hook up to building HVAC systems. Yuan says that while his new system could be designed as a small reactor, for now “we are trying to scale up the technology for direct air capture.” They plan to use the algae grown with the captured CO2 for fuels but also for other more sustainable and cost-effective uses.